Introduction to AI in Financial Markets
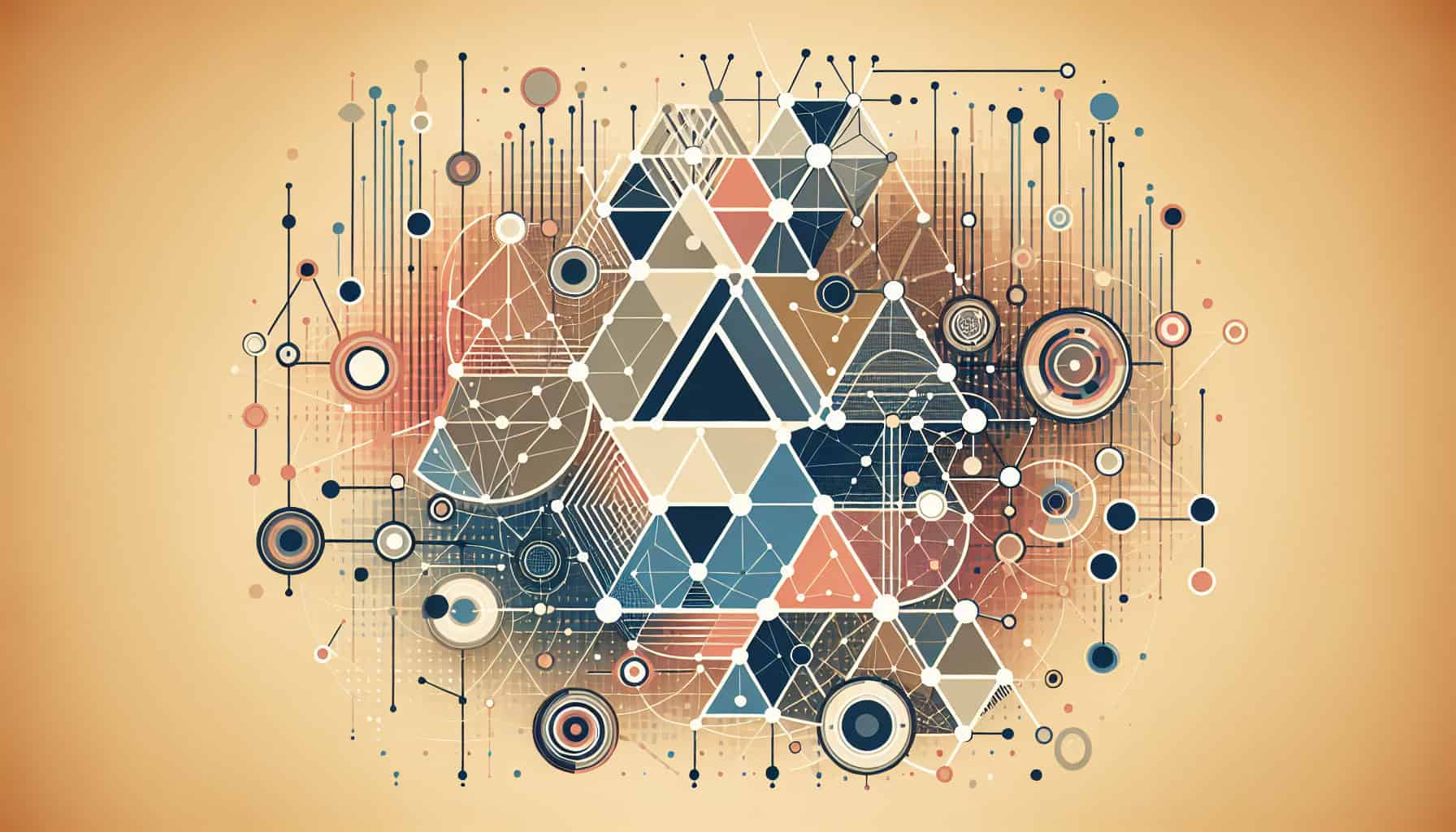
Introduction to AI in Trading
The fusion of artificial intelligence (AI) with the financial sector has revolutionized how markets operate, paving the way for more efficient, secure, and intelligent trading. This introduction provides a glimpse into the burgeoning role of AI within the trading landscape.
The Rise of AI in Finance
AI’s ascent in the financial markets has been nothing short of meteoric. Financial institutions have recognized AI’s potential in streamlining operations, enhancing customer experiences, and bolstering bottom lines. Business Insider forecasts monumental cost savings for banks and financial firms, with AI applications potentially saving $447 billion by 2023, and about $1 trillion by 2030 as reported by the University of San Diego. The market value of AI in finance was at an impressive $9.45 billion in 2021 and is expected to burgeon at a compound annual growth rate (CAGR) of 16.5 percent leading up to 2030.
Key AI Technologies in Trading
AI in trading leverages a variety of technologies, each contributing uniquely to the field:
-
Machine Learning and Predictive Analytics: Machine learning algorithms are at the heart of AI’s prowess in trading, aiding in predictive analytics for financial markets and volatility prediction. These models learn from historical data to forecast market trends and identify profitable trading opportunities.
-
Natural Language Processing (NLP): NLP is instrumental in automated news analysis and market sentiment analysis, enabling systems to interpret and quantify qualitative information like news articles and social media posts.
-
Neural Networks: These are particularly adept at price prediction, thanks to their ability to model complex, non-linear relationships in financial data.
-
Reinforcement Learning: This is an area of machine learning that’s particularly useful in developing trading strategies, where the algorithm learns by interacting with a financial environment to achieve optimal decision-making.
-
Evolutionary Algorithms: Used in portfolio management, these algorithms simulate the process of natural selection to generate robust investment strategies.
-
Supervised and Unsupervised Learning: Both these types of learning are integral in market analysis, whether it’s classifying different market regimes or uncovering hidden structures in market data.
-
Deep Learning: This subset of machine learning is crucial for algorithmic trading, enabling more accurate and complex models that can detect subtle patterns in large datasets.
-
Feature Engineering: This process enhances trading models by identifying and selecting the most relevant variables or creating new features that improve model performance.
Traders, investors, and financial institutions are harnessing these AI technologies not only to augment their existing strategies but also to innovate and remain competitive in a rapidly evolving financial landscape. As AI continues to advance, the financial markets are set to become even more sophisticated, efficient, and adaptive.
The Impact on Financial Markets
Artificial Intelligence (AI) is revolutionizing the financial arena, offering unprecedented enhancements in efficiency and risk management. As AI technologies are harnessed for various applications within the sector, their impact is profound, leading to significant operational improvements and cost reductions.
Operational Efficiencies and Cost Savings
AI’s integration into financial markets has led to considerable operational efficiencies. According to the University of San Diego, AI applications could save banks and financial institutions up to $447 billion by 2023. This reduction in costs is largely attributed to AI’s ability to automate labor-intensive tasks, which minimizes manual errors and reduces the time spent on processing tasks. As Ocrolus suggests, AI-powered document automation alone can streamline data entry, document sorting, and verification tasks.
One compelling illustration of AI’s cost-saving potential is in the automation of data entry and document processing. By automating these tasks, financial institutions can significantly cut operational expenses tied to manual labor:
Process | Traditional Cost | AI-Enhanced Cost Savings |
---|---|---|
Data Entry | X amount | Reduced by Y% |
Document Processing | X amount | Reduced by Y% |
*Note: X and Y would be specific numerical values reflecting cost and savings, respectively.
In addition, AI’s real-time data analysis and predictive modeling capabilities enable financial institutions to make swifter and more informed decisions, optimizing business strategies and scaling operations efficiently in the ever-dynamic financial landscape.
Enhancing Risk Management and Fraud Detection
Risk management and fraud detection are two areas where AI is making a substantial difference. With 70% of financial firms employing machine learning to adjust credit scores and detect fraud, as highlighted by the University of San Diego, AI algorithms have proven adept at analyzing vast data sets in real-time to identify patterns and anomalies indicative of fraudulent activities.
The use of AI in detecting fraud is not only proactive in identifying risks but also enhances the overall security of financial transactions. The following table showcases the types of fraud AI can detect and the potential reduction in fraud cases:
Fraud Type | Pre-AI Detection Rate | Post-AI Detection Rate Improvement |
---|---|---|
Credit Card Fraud | X% | Improved by Y% |
Insurance Fraud | X% | Improved by Y% |
*Note: X and Y would be specific numerical values reflecting the detection rate and improvement, respectively.
Moreover, AI’s predictive analytics further fortify risk management efforts by assessing risks and identifying investment opportunities, contributing to the overall stability and security of financial markets. For more insights into how AI transforms risk management in trading, readers can explore ai risk management trading.
The deployment of AI in financial markets is not without its challenges, but the benefits it brings to operational efficiency and fraud detection are clear and substantial. As AI continues to evolve, it promises to further reshape financial markets, offering both opportunities and new considerations for all market participants. For a deeper dive into the role of AI in trading, readers can explore topics such as neural networks price prediction and deep learning algorithmic trading.
AI’s Role in Personalizing Finance
Artificial Intelligence (AI) is revolutionizing the financial sector by offering personalized services that were once thought impossible at scale. AI’s ability to process vast quantities of information quickly and discern intricate patterns in data facilitates more insightful decision-making and tailored financial experiences for customers.
Customizing Banking Services
Financial institutions are now harnessing the power of AI to deliver personalized banking services to their clients. AI-driven systems can analyze customer data, including transaction history, preferences, and behavior, to offer bespoke services that cater to individual needs. This personalization extends to product recommendations, financial advice, and even predictive account services, ensuring that customers receive a banking experience that feels both unique and relevant to their financial situation.
Furthermore, AI technologies are enhancing customer engagement by ensuring that relationship-based services are available precisely when customers need them the most, creating a sense of reliability and trust between the client and the institution (Google Cloud).
One of the transformative technologies in customizing banking services is the deployment of AI chatbots. These virtual assistants provide immediate, 24/7 customer service, handling inquiries and simple tasks, which allows human customer service representatives to focus on more complex issues, thereby improving overall efficiency and customer satisfaction.
AI-Driven Investment Strategies
In the realm of investments, AI is playing a pivotal role in developing sophisticated strategies that were previously unattainable through traditional methods. Machine learning, a subset of AI, utilizes algorithms that learn and improve autonomously by analyzing large datasets. This capacity for learning enables the creation of dynamic investment models that adapt to market changes in real-time (Google Cloud).
AI-driven investment strategies can include a range of approaches, such as:
- Predictive analytics for forecasting market trends and movements (predictive analytics financial markets).
- Utilizing neural networks for price prediction to anticipate asset price fluctuations.
- Applying reinforcement learning to optimize trading algorithms based on reward feedback mechanisms.
- Conducting sentiment analysis through natural language processing (NLP) to gauge the market sentiment from news articles, social media, and financial reports.
AI’s ability to process and analyze an enormous volume of market data provides investors with valuable insights, enabling them to make more informed decisions. The result is a more personalized investment experience that is attuned to the unique risk profile and financial goals of each investor.
AI integration into the financial markets is not only reshaping how institutions interact with their clients but also how individuals approach their personal finance and investment decisions. By leveraging AI’s capabilities, financial services are becoming more intuitive, efficient, and customer-centric, paving the way for a future where finance is increasingly personalized and accessible to all.
Addressing the Challenges
The integration of artificial intelligence (AI) and machine learning (ML) into the financial markets revolutionizes trading but also introduces a series of challenges that need to be carefully managed. Two primary areas of concern are the regulatory and ethical considerations, and the mitigation of bias while ensuring privacy.
Regulatory and Ethical Considerations
The rapid advancement of AI in trading has outpaced the establishment of regulatory guidelines, leading to a lack of clear direction for oversight (EY). Regulatory bodies have raised concerns regarding AI/ML applications, such as the potential for embedding bias in credit decision algorithms or the dissemination of misleading information by AI-driven chatbots. Issues like data privacy, cybersecurity, and the transparency of AI models are under increasing scrutiny, especially with the advent of generative AI.
To address these concerns, it is essential for regulatory frameworks to evolve in tandem with AI advancements. This means that financial institutions must engage in ongoing dialogue with regulators to ensure compliance and to contribute to the development of standards that adequately reflect the capabilities and risks associated with AI in finance.
Furthermore, ethical considerations must be at the forefront of AI deployment in financial services. This includes a commitment to fairness, accountability, and transparency in AI systems. Financial institutions should develop and adhere to ethical guidelines that address the responsible use of AI, ensuring that these systems do not perpetuate existing biases or unfair practices.
Mitigating Bias and Ensuring Privacy
Bias in AI systems can lead to unfair outcomes, particularly in areas like credit scoring or customer segmentation. These biases can stem from skewed datasets or flawed algorithmic design. The “black box” nature of some AI applications can make it difficult to trace how these biases occur, which complicates efforts to remove them.
To mitigate this issue, it’s crucial to implement robust data governance practices that include careful curation and analysis of training data. Deploying explainable AI (XAI) techniques can improve transparency, making it easier to identify and correct biases. Additionally, diversity in the teams developing AI solutions can help reduce the risk of unconscious biases being coded into algorithms.
Privacy is another significant concern as AI systems often require vast amounts of personal data to function effectively. Ensuring data privacy means adhering to regulations such as GDPR and other local data protection laws. It also involves employing advanced cybersecurity measures to protect against unauthorized access and potential breaches.
Financial institutions must be vigilant in their use of AI to avoid infringing upon individual privacy. This includes transparent data collection policies, secure data storage solutions, and clear communication with clients about how their data is being used.
As AI continues to shape the financial markets, addressing these challenges is imperative for sustainable growth and customer trust. By fostering regulatory cooperation, adhering to ethical standards, eliminating biases, and prioritizing privacy, the finance industry can harness the full potential of AI while maintaining the integrity of the markets. For more on how AI is transforming trading practices, explore our articles on neural networks price prediction and reinforcement learning trading.
The Future of AI in Trading
The intersection of artificial intelligence (AI) and finance is poised to redefine the landscape of trading. In this section, we will explore what the future holds for AI in finance and how entities in the financial sector can prepare for the upcoming AI-driven transformation.
Predictions for AI in Finance
Artificial intelligence is at the forefront of innovation in financial markets, with predictions indicating significant cost savings and efficiency gains. By 2023, AI applications are expected to save banks and financial institutions a staggering $447 billion, with the potential to save the banking industry about $1 trillion by 2030. The market value of AI in finance, which was estimated at $9.45 billion in 2021, is projected to grow exponentially, at a rate of 16.5 percent by 2030 (Source).
AI is set to revolutionize several domains within the financial sector, enhancing operational efficiencies in risk management, trading, insurance underwriting, and more (Toptal). From machine learning trade execution to predictive analytics in financial markets, the applications are diverse and powerful.
One of the most promising areas of AI in trading is the capacity to optimize and automate processes. For instance, AI-powered document automation can reduce processing time and minimize manual errors, significantly cutting down operational costs associated with manual labor (Ocrolus). Additionally, AI serves as a potent tool in fraud detection, leveraging algorithms that can sift through vast amounts of data to identify irregular patterns indicative of fraudulent activities, thereby reinforcing risk management strategies (Ocrolus).
Furthermore, AI has the potential to deliver real-time data analysis and insights, facilitating quicker and more informed decisions across financial markets. This translates to faster decision-making and increased scalability, critical in today’s fast-paced financial environment.
Preparing for AI-Driven Financial Services
Preparation for AI-driven financial services involves several key steps. Financial institutions must first recognize the multifaceted role of AI, which includes personalizing services, managing risk and fraud, enabling transparency and compliance, and automating operations to reduce costs.
Embracing AI requires a shift in mindset toward data-driven decision making. AI’s ability to process information rapidly and uncover patterns can lead to more insightful risk modeling, compliance management, and overall, smarter financial services.
For successful implementation, institutions should focus on:
- Adopting best practices for AI adoption in trading, ensuring the technology works autonomously and responsibly.
- Addressing the challenges of mitigating bias and ensuring privacy in AI systems.
- Overcoming barriers to integration, including technological infrastructure upgrades and employee training.
- Leveraging AI to automate workflows and empower decision-making, ultimately enhancing customer engagement and service delivery (Google Cloud).
The future of AI in trading is not just about technological advancement but also about the strategic integration of this technology into existing financial frameworks. By staying informed about future AI trading trends and preparing for the changes AI will bring, the financial sector can position itself at the forefront of this exciting wave of innovation.
Implementing AI in Trading
The integration of AI in trading has been transforming the financial markets, optimizing operations, and offering new insights and efficiencies. Below are the best practices for adoption and strategies for overcoming barriers to integration, ensuring a smooth transition into the AI-enhanced trading environment.
Best Practices for Adoption
When adopting AI technologies in trading, it’s essential to follow practices that can lead to successful implementation:
- Start with Clear Objectives: Define what you want to achieve with AI, be it improved risk management, better trade execution, or enhanced predictive analytics.
- Data Quality and Management: Ensure you have access to high-quality data, as AI models are only as good as the data they are trained on. Prioritize data cleaning and preprocessing to improve model accuracy.
- Infrastructure Investment: Establish a robust IT infrastructure capable of supporting AI algorithms, including sufficient computational power and storage.
- Talent Acquisition: Hire or train experts in AI, data science, and machine learning to develop and maintain your AI systems.
- Risk Management: Implement AI with a thorough understanding of the potential risks, including market risks and ethical considerations.
- Regulatory Compliance: Stay informed about the regulatory landscape and ensure that AI solutions are compliant with all relevant trading regulations.
- Testing and Validation: Rigorously test AI models using historical and real-time data to validate their effectiveness before full-scale deployment.
- Continuous Learning and Adaptation: AI models require ongoing monitoring and fine-tuning to adapt to market changes and maintain performance.
For insights on specific AI applications in trading, explore our detailed guides on neural networks price prediction, reinforcement learning trading, and nlp market sentiment analysis.
Overcoming Barriers to Integration
Integrating AI into trading operations can come with its set of challenges. Here’s how to address common barriers:
- Cultural Resistance: Educate and train staff to embrace AI by highlighting its benefits and addressing concerns around job displacement.
- Complexity of Models: Simplify AI models as much as possible and use explainable AI to make their decisions understandable to stakeholders.
- Data Privacy: Use advanced encryption and anonymization techniques to protect sensitive data, aligning with privacy regulations and ethical standards.
- Cost Concerns: Highlight the long-term cost savings and ROI that AI can bring by automating tasks and improving decision-making.
- Integration with Existing Systems: Develop a phased integration plan that allows AI systems to work alongside existing technologies without disruption.
For further exploration of AI in financial markets, consider reading about ai risk management trading and evolutionary algorithms portfolio management. Additionally, our resources on ai high frequency trading strategies and predictive analytics financial markets can provide specialized knowledge for those looking to delve deeper into AI’s potential.
By adhering to these best practices and overcoming integration barriers, financial institutions and traders can leverage the full potential of AI to revolutionize their trading strategies and operations.