Unlocking the Secrets: Case Studies of Algorithmic Trading Success
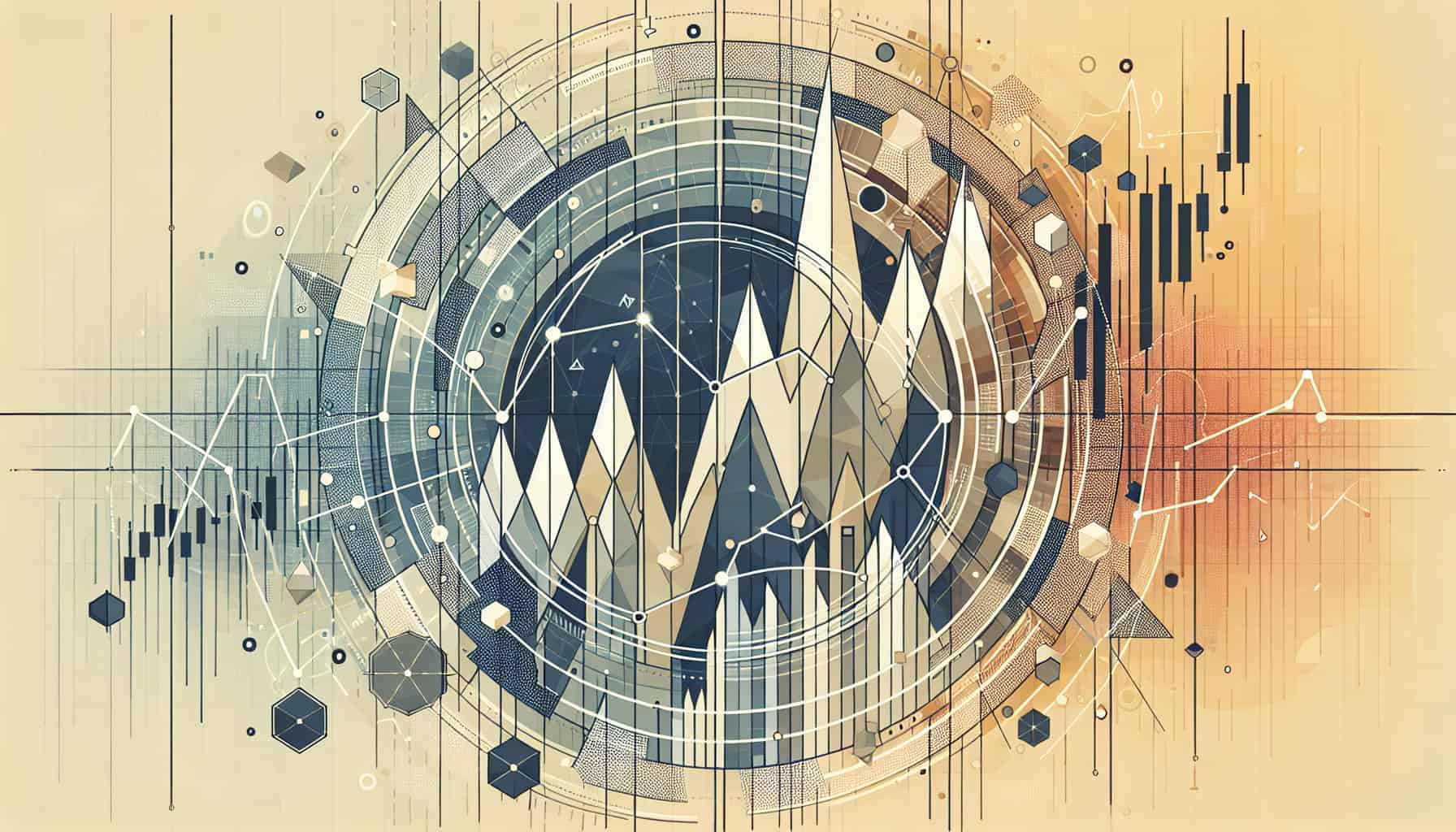
Understanding Algorithmic Trading
Algorithmic trading, also known as algo trading, has become a significant force in the financial markets, offering a range of advantages for investors and institutions alike.
Basics of Algo Trading
Algo trading involves the use of computer programs that follow a defined set of instructions, or algorithms, to place trades. The aim is to take advantage of various market factors at speeds and frequencies that are beyond human capabilities. Algorithmic strategies can range from simple to complex and can be applied across various asset classes, including equities, forex, and cryptocurrencies.
The foundations of algorithmic trading lie in the systematic analysis of market data and the execution of trades based on precise criteria. This can include a variety of signals such as price movements, technical indicators, and statistical arbitrage opportunities. Algorithms are meticulously backtested with historical data to evaluate their efficacy before being deployed in live markets.
Key Features and Advantages
One of the primary advantages of algorithmic trading is the ability to execute trades at a speed and precision that is unattainable for human traders. As explained by Faster Capital, trades can be completed in milliseconds, capturing fleeting market opportunities that could otherwise be missed.
Another key benefit is the elimination of emotional decision-making and human error. Algorithms operate on a set of predefined rules and are not swayed by psychological factors, ensuring disciplined and consistent trading (Faster Capital).
Diversification is another aspect where algo trading shines. Traders can simultaneously manage multiple positions across different markets, thereby spreading risk and increasing the potential for returns (Faster Capital).
Algorithms also allow for extensive backtesting using historical data. This process helps traders understand the potential risks and returns of their strategies, enabling them to refine and optimize their algorithms.
High-frequency trading, a subset of algo trading, exemplifies the importance of speed, with firms executing trades in microseconds to capitalize on market inefficiencies (Investopedia).
These features contribute to the success stories within the realm of algorithmic trading, such as Renaissance Technologies’ impressive returns, highlighting the potential for substantial gains when leveraging advanced trading algorithms (uTrade Algos).
For those interested in the history of algorithmic trading or seeking to understand the basic algorithmic trading models, there are extensive resources available to help navigate this complex field. As technology evolves, the future technologies impacting algorithmic trading will continue to redefine the landscape of the financial markets.
Algorithmic Trading in Action
The practical applications of algorithmic trading demonstrate why it has become a staple in modern finance. Traders utilize algorithms to execute trades with unparalleled speed, manage risk efficiently, and continuously refine strategies for optimal performance.
Speed and Precision in Execution
Algorithmic trading excels in executing trades with incredible speed and precision. Trades can be carried out in milliseconds, capitalizing on fleeting market opportunities that would be impossible to exploit manually (Faster Capital). High-frequency trading firms, for instance, operate on a timescale of microseconds, showcasing the upper echelons of speed achievable.
The absence of human intervention in the execution process eliminates the potential for error, ensuring trades align precisely with the strategy’s specifications. As a result, algorithmic trading fosters a more disciplined approach to market engagement, insulated from emotional decision-making.
Aspect | Benefit |
---|---|
Speed | Executes trades in milliseconds to microseconds |
Precision | Eliminates human error in trade execution |
Discipline | Follows predefined rules without emotional influence |
Diversification and Risk Management
Algorithmic trading enables extensive diversification, allowing simultaneous trades across various instruments and markets. This broadens the risk distribution and contributes to a more resilient investment portfolio (Faster Capital).
Effective risk management is a cornerstone of successful trading, and algorithms can be programmed with risk parameters to limit potential losses. They can automate stop-loss orders and adjust positions based on real-time market data, helping to mitigate adverse market movements.
Backtesting and Performance Optimization
A critical advantage of algorithmic trading is the ability to backtest strategies using historical data. This process evaluates how an algorithm would have performed in the past, informing adjustments for improved future performance (Faster Capital).
Backtesting provides a quantitative measure of a strategy’s viability, highlighting strengths, weaknesses, and potential areas for optimization. Continuous refinement through backtesting leads to more robust and adaptable strategies that can withstand market complexities.
Stage | Objective |
---|---|
Backtesting | Assess historical performance |
Optimization | Refine strategy for future conditions |
The operational prowess of algorithmic trading is undeniable, as evidenced by case studies: algorithmic trading success stories like Renaissance Technologies and Two Sigma. As traders navigate the algorithmic trading software basics, they gain access to sophisticated tools that can lead to consistent profitability and strategic advantage in the global landscape of algorithmic trading. For novices, understanding the basic algorithmic trading models is an essential first step, while seasoned professionals continually seek ways to integrate future technologies impacting algorithmic trading into their systems.
Case Studies of Success
The landscape of finance has been revolutionized by algorithmic trading, with numerous firms and funds demonstrating extraordinary success. These case studies highlight the triumphs of some of the most successful entities in algorithmic trading, showcasing the potential of automated systems in generating impressive financial returns.
Renaissance Technologies’ Medallion Fund
Renaissance Technologies, particularly its Medallion Fund, stands as a paragon of algorithmic trading achievement. From 1994 through mid-2014, the Medallion Fund has generated annual returns averaging 71.8% before fees, a figure that is nearly unmatched in the trading industry. This fund, known for its secrecy and selective investor base, attributes its success to complex mathematical models and sophisticated algorithms that can predict market changes with incredible accuracy.
The Medallion Fund is an excellent example of how quantitative analysis in algo trading can yield exceptional results. The fund’s focus on statistical arbitrage and other strategies helps to capitalize on market inefficiencies. For those interested in the history and evolution of such trading strategies, further reading can be found in the article on the history of algorithmic trading.
Virtu Financial’s Consistent Profits
Another standout in the realm of high-frequency trading is Virtu Financial. In 2018, the firm reported a remarkable record, with only one losing day out of 1,238 trading days. Such consistency is a testament to the power of algorithmic trading methods, which enable a level of precision and speed that human traders cannot match. Virtu Financial’s success underscores the importance of speed in algorithmic trading, as well as the firm’s robust risk management strategies and real-time analysis capabilities.
For traders looking to understand the mechanics behind such success, exploring basic algorithmic trading models can provide valuable insights into the foundational elements that contribute to consistent profitability.
Two Sigma’s Quantitative Edge
Two Sigma Investments has carved out a significant presence in the world of algorithmic trading by managing over $50 billion in assets as of 2015. Founded in 2001, Two Sigma’s quantitative edge is derived from the use of advanced data analysis techniques, machine learning, and artificial intelligence to inform its trading decisions. This case clearly illustrates how big data and machine learning play pivotal roles in modern algorithmic trading strategies.
Two Sigma’s approach also emphasizes the significance of evaluating market data for algorithmic trading, using a vast array of information sources to gain a competitive edge in various financial markets, including equity and forex markets.
These case studies provide just a glimpse into the success that can be achieved through algorithmic trading. While these entities have demonstrated exceptional results, it is crucial for investors and traders to remain aware of the challenges and risks associated with this type of trading, as well as the regulatory framework that governs it. By learning from these success stories and understanding the complexities of algorithmic trading, individuals and firms can strive towards achieving their own trading goals.
Challenges and Risks
While algorithmic trading has revolutionized the finance sector with its speed and precision, it brings its own set of challenges and risks. Navigating these complexities is vital for traders looking to harness the power of algorithms in the markets.
Market Volatility and Stop-Loss Strategies
Market volatility represents one of the most significant challenges in algorithmic trading. Rapid fluctuations can lead to substantial losses in a short amount of time. To combat this, traders implement stop-loss orders, which automatically sell a security when it reaches a certain price, thus capping potential losses. By setting these thresholds, traders can mitigate risks associated with sudden market downturns. For a deeper understanding of order types in algorithmic trading, explore our article on understanding order types in algorithmic trading.
Market Condition | Stop-Loss Strategy | Outcome |
---|---|---|
Rapid Price Decline | Stop-Loss Order | Limits Losses |
Sudden Market Spike | Trailing Stop-Loss | Secures Profits |
Source: Faster Capital
Technical Failures and Redundancy Measures
Algorithmic trading relies heavily on technology, making it susceptible to technical failures like software glitches, hardware malfunctions, or connectivity issues. These can disrupt trading strategies, leading to potential financial loss. Redundancy measures such as backup systems and alternative internet connections are essential to ensure continuity. More on preventing such failures can be found in our guide to algorithmic trading software basics.
Over-Optimization and Real-Time Performance
Over-optimization occurs when an algorithm is excessively fine-tuned to historical data, causing it to underperform in live trading. To prevent this, traders utilize techniques such as out-of-sample testing and walk-forward analysis, which assess the algorithm’s effectiveness with new data sets. This helps ensure that the algorithm remains robust under various market conditions. Readers can learn more about this in our section on common mistakes in algorithmic trading.
Data Quality and Decision Making
The success of algorithmic trading is heavily reliant on the quality of input data. Inaccurate or incomplete data can lead to poor decision-making and significant financial loss. Traders must employ rigorous data cleaning and validation processes to ensure the integrity of the data used by their algorithms. An in-depth look at data evaluation can be found here: evaluating market data for algorithmic trading.
Algorithmic trading, while offering numerous benefits, also demands a high level of diligence in managing the associated risks and challenges. Understanding these complexities is crucial for traders who wish to leverage algorithms for market success. It is also important to stay informed about the regulatory framework for algorithmic trading to ensure compliance and avoid legal pitfalls. As technology continues to evolve, staying ahead of these challenges will remain a top priority for traders. For insights into how technology will shape the future of this field, be sure to read about future technologies impacting algorithmic trading.
Regulatory Landscape
The regulatory environment for algorithmic trading is complex and stringent. It requires traders to stay well-informed and diligent to ensure full compliance with the rules and regulations governing financial markets.
Compliance in Algo Trading
For participants in the algorithmic trading space, adherence to regulatory compliance is not optional but mandatory. Regulatory bodies have instituted various rules to ensure fair and transparent markets, protect investors, and maintain market integrity. Compliance with such regulations helps in averting substantial fines and legal actions that could arise from non-compliance.
Algorithmic trading, like any trading activity, must align with all relevant regulations. Traders can collaborate with regulatory experts and legal professionals to ensure their trading algorithms are compliant, as failure in doing so can lead to stringent consequences (FasterCapital). For example, regulatory frameworks like the Markets in Financial Instruments Directive (MiFID II) in the European Union have been specifically designed to heighten transparency and reinforce investor protection in the financial markets. These frameworks require traders to report their algorithmic trading activities and ensure their systems are resilient, have sufficient capacity, and are subject to appropriate trading thresholds and limits.
For a deeper understanding of the regulatory framework for algorithmic trading, one can delve into the historical and current regulations that shape the practices of algo trading today.
Legal Considerations and Best Practices
Legal considerations are integral to the operation of algorithmic trading. Traders should seek counsel from legal professionals who specialize in financial markets to navigate the complexities of compliance. This legal guidance is critical to understand the nuances of laws and regulations that impact algorithmic trading activities.
Best practices in algorithmic trading compliance include:
- Thoroughly understanding the regulatory requirements in the jurisdictions where the trading occurs.
- Maintaining accurate and comprehensive records of all trading activities.
- Implementing robust risk management systems and controls to prevent market manipulation, such as spoofing and layering.
- Regularly reviewing and updating algorithms to ensure they operate as intended and within legal frameworks.
- Conducting rigorous backtesting and performance optimization while being mindful of the risks associated with over-optimization.
By adhering to these best practices, traders can not only comply with regulations but also reinforce the legitimacy and reliability of their trading strategies. It’s also worthwhile to stay updated on the global landscape of algorithmic trading to comprehend how different regions approach regulation in this domain.
Navigating the regulatory landscape of algorithmic trading can be daunting, but it is an essential aspect of implementing successful trading strategies. With the financial sector continually evolving, both regulatory bodies and traders must adapt to new challenges and changes, ensuring markets remain just and lawful for all participants.
Future of Algorithmic Trading
The landscape of algorithmic trading is constantly evolving, with technological advancements, the incorporation of machine learning, and the utilization of big data significantly impacting the financial sector. As we look ahead, these elements are poised to further revolutionize the way trading strategies are devised and executed.
Advancements in Technology
Technological progress has been a driving force behind the development of algorithmic trading. Firms engaged in high-frequency trading now complete trades in microseconds, showcasing their capacity to process large volumes of data and execute trades at unprecedented speeds (Investopedia). This blistering pace is likely to increase as advancements in computing power and network infrastructure continue to emerge.
The future of algorithmic trading technology may involve quantum computing, distributed ledgers, and enhanced encryption methods, which will further expedite trade execution and improve security. As these technologies become more accessible, they will enable a wider range of market participants to implement advanced trading strategies.
Role of Machine Learning and Big Data
Machine learning algorithms are transforming algorithmic trading by offering adaptability and enhanced predictive capabilities. These algorithms can learn from a myriad of market scenarios and adjust their strategies in real-time to optimize performance. The use of SEDOL codes, which are unique identifiers assigned to securities for efficient organization, enhances the precision of these machine learning models by facilitating the analysis of historical data (FasterCapital).
Big data analytics plays a complementary role by allowing traders to sift through vast quantities of market information. This leads to more accurate and data-driven decision-making, which is critical in developing successful trading strategies (FasterCapital). The symbiosis of machine learning and big data is expected to continue growing, with sophisticated analytical tools processing an ever-expanding data universe to deliver refined insights.
Impact on the Financial Sector
The integration of cutting-edge technology and data science in algorithmic trading is having a profound impact on the financial industry. As these tools become more advanced and widespread, the financial sector is seeing a shift towards more quantitative and systematic approaches to trading.
Algorithmic trading firms that successfully leverage these developments tend to outperform traditional trading methods, leading to a competitive edge that is reshaping market dynamics. Regulatory bodies are also taking note, with an increased focus on overseeing these technologies to ensure market stability and fairness (regulatory framework for algorithmic trading).
In conclusion, the future of algorithmic trading is intertwined with the continuous innovation in technology, machine learning, and data analysis. These components will not only define the trading strategies of tomorrow but will also influence the structure and operation of the entire financial landscape. As we move forward, traders and financial institutions will need to stay abreast of these changes to remain competitive and harness the full potential of algorithmic trading.