Unveiling the Secrets: Navigating the Global Landscape of Algorithmic Trading
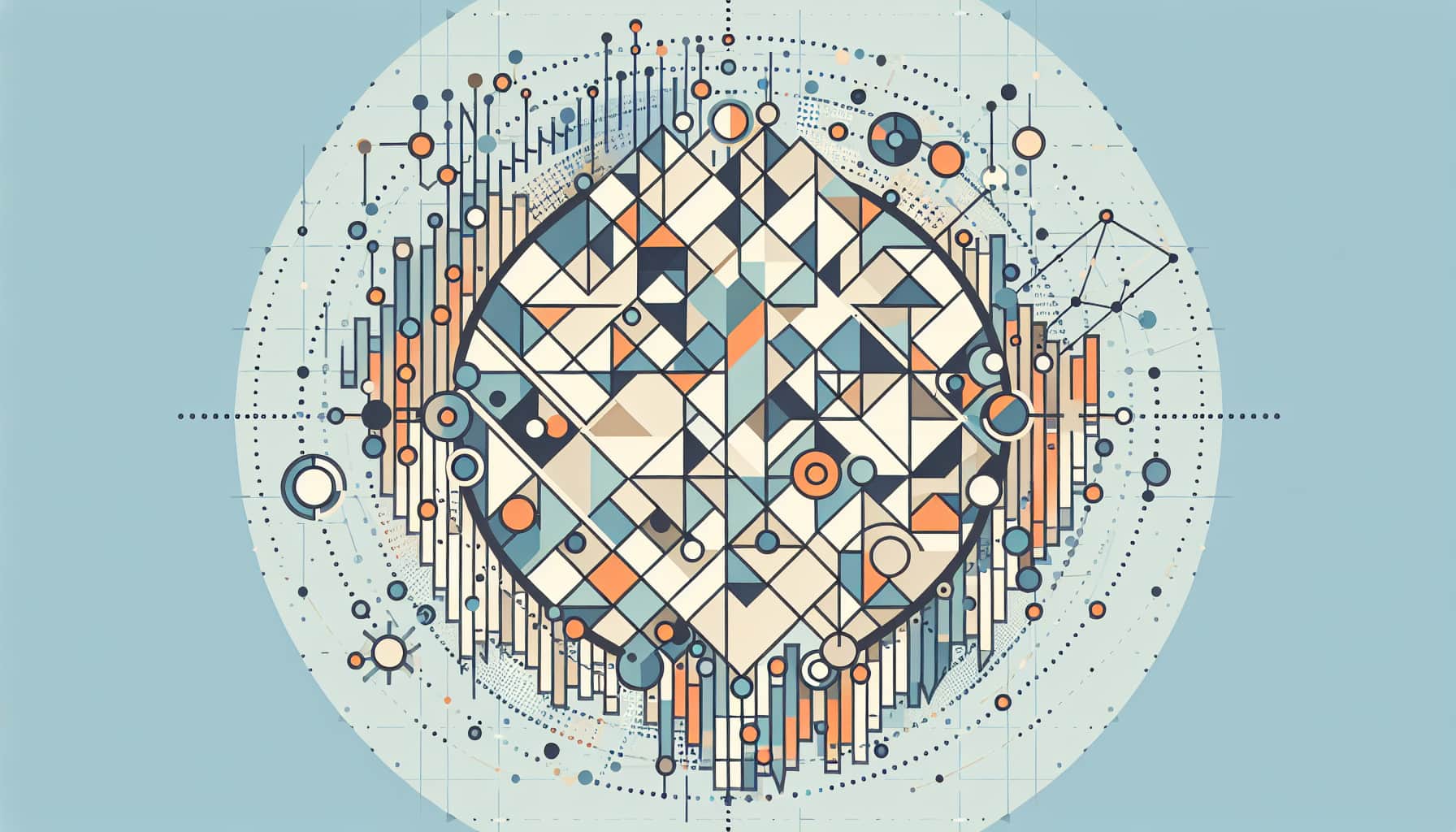
Understanding Algorithmic Trading
In the dynamic world of finance, algorithmic trading has emerged as a transformative force shaping the very fabric of the markets. This section delves into the essence of algorithmic trading and traces its advancement over time, laying the groundwork for a comprehensive exploration of the global landscape of algorithmic trading.
What is Algorithmic Trading?
Algorithmic trading, also known as algo trading, is the automated execution of trades on financial markets using advanced computer algorithms and mathematical models. This data-driven approach to trading has become increasingly popular among institutional investors and high-frequency traders due to its ability to execute trades with high speed and precision. By minimizing the impact of market volatility and reducing the influence of human emotions on trading decisions, algorithmic trading has revolutionized how market participants engage with financial markets.
The use of algorithms enables traders to process orders faster and more accurately, capitalizing on small price discrepancies across multiple markets. The efficiency and speed at which trades can be executed have now reached a point where some transactions are completed in mere fractions of a second.
For individuals keen on diving deeper into the mechanics of these systems, algorithmic trading software basics provide a starting point for understanding the technological underpinnings of algo trading platforms.
The Evolution of Trading Systems
The inception of algorithmic trading can be traced back to the 1980s when traders began to leverage the power of computers to automate aspects of the trading process. Initially, these systems were rudimentary, executing simple trades based on price thresholds. However, as technology advanced, these algorithms evolved, becoming capable of analyzing vast datasets and executing complex trading strategies.
With the rise of High-Frequency Trading (HFT), characterized by ultra-fast trade execution, the landscape of the financial markets has dramatically changed. HFT often involves executing trades within microseconds, contributing significantly to market liquidity and reducing trading costs. Moreover, the advent of artificial intelligence (AI) in algorithmic trading has allowed for the analysis and learning from extensive data sets, adapting strategies in real-time, albeit with a note of caution regarding the system’s unpredictability and potential for error.
The historical trajectory of these trading systems is not just a matter of technical evolution but also reflects shifts in market dynamics and regulatory frameworks. Individuals can explore the history of algorithmic trading to gain a more nuanced understanding of its development over time.
As we stand on the cusp of a new era in trading, the continued evolution of algorithmic systems paints an intriguing picture of the future, where technology and human expertise converge to forge new pathways in the financial markets.
The Growth of Algorithmic Trading
The global landscape of algorithmic trading has experienced remarkable expansion, transforming the way financial markets operate. This section assesses the market size and growth projections, along with a regional analysis of algorithmic trading adoption.
Market Size and Growth Projections
Algorithmic trading, a method that automates trading using pre-programmed instructions, has shown substantial growth in market size. In 2023, the market was valued at USD 15.76 billion and is anticipated to accelerate at a Compound Annual Growth Rate (CAGR) of 10.6%, potentially reaching USD 31.90 billion by the year 2030.
The driving forces behind this growth include technological advancements, an uptick in demand for cloud-based solutions, and the increasing adoption of algorithmic trading by institutional investors. These factors contribute to the enhanced speed, precision, and cost-effectiveness of trade execution that algorithmic trading offers.
Year | Market Size (USD Billion) | CAGR (%) |
---|---|---|
2023 | 15.76 | – |
2030 (Projected) | 31.90 | 10.6 |
Regional Market Analysis
Geographically, North America stands as the frontrunner in the algorithmic trading market. This is attributed to significant investments in trading technologies and an established financial infrastructure. Meanwhile, the Asia Pacific region is emerging as a rapidly expanding market, driven by an increased adoption of advanced trading solutions.
The Algorithmic Trading market’s components include a spectrum of solutions and services, with solutions taking the lead due to their role in streamlining transaction costs and facilitating efficient trade executions. The market covers a diverse range of trading types, from Foreign Exchange (FOREX) and Stock Markets to Exchange-Traded Funds (ETFs), Bonds, Cryptocurrencies, and more.
Region | Growth Drivers |
---|---|
North America | Advanced trading technologies, robust financial sector |
Asia Pacific | Growing adoption of algorithmic trading, technological investments |
Despite the promising trajectory, the algorithmic trading market faces challenges, including a lack of risk evaluation capabilities and regulatory hurdles. To navigate these complexities, individuals and organizations may explore resources such as basic algorithmic trading models, algorithmic trading software basics, and regulatory framework for algorithmic trading.
The growth of algorithmic trading is reshaping the global financial markets, offering new opportunities for efficiency and effectiveness in trade executions. As the market continues to evolve, participants ranging from beginner traders to regulatory professionals are encouraged to stay informed about the latest technological advancements and market trends to capitalize on the potential of algorithmic trading.
Key Components and Technologies
The global landscape of algorithmic trading is built upon a foundation of sophisticated components and cutting-edge technologies. These elements work collectively to enable the rapid, efficient, and intelligent execution of trades across financial markets.
Solutions and Services Breakdown
Algorithmic trading encompasses various services and solutions that cater to different aspects of the trading process. These can range from trade execution services to comprehensive risk management solutions.
- Trade Execution Services: These services utilize algorithms to automate the buying and selling of securities at optimal prices and times, often with minimal human intervention.
- Risk Management Solutions: Algorithms can also help traders and institutions manage risk by monitoring market conditions and adjusting positions in real-time.
- Data Analysis Services: Access to real-time and historical market data is crucial for algorithmic trading. Services that provide data analysis support traders in making informed decisions.
- Infrastructure Providers: Reliable infrastructure is vital for algorithmic trading. This includes network connectivity, data storage, and computational power.
- Compliance and Surveillance: As the regulatory environment tightens, services that ensure compliance and monitor for suspicious activities become increasingly important.
Each of these services plays a crucial role in the ecosystem of algorithmic trading, and understanding their nuances is essential for anyone involved in the industry. For more insights on the basic algorithmic trading models, readers can explore further.
Role of Advanced Technologies
The growth and sophistication of algorithmic trading are largely driven by advancements in technology. These technologies have not only reshaped the trading landscape but have also introduced new capabilities and efficiencies.
- Machine Learning Algorithms: By leveraging machine learning, trading algorithms can adapt to new data and patterns, resulting in more dynamic and intelligent decision-making processes.
- Big Data Analytics: The ability to process and analyze vast amounts of data in real-time has allowed traders to gain deeper insights and make more accurate predictions.
- High-Speed Computing: With trades being executed in fractions of a second, high-speed computing power is essential for maintaining a competitive edge.
- Cloud Computing: Cloud infrastructure offers scalable and cost-effective resources for algorithmic traders, facilitating access to computational power and data storage.
- Quantitative Analysis: Quantitative methods are used to develop algorithms that can identify trading opportunities based on statistical data.
Advanced technologies such as machine learning algorithms, big data analytics, and cloud computing enhance the capabilities of algorithmic trading, allowing for the real-time processing of vast data amounts for informed trading decisions. These technological advancements have not only streamlined operations but have also contributed to improved market liquidity and efficiency, benefiting all market participants (Acumen Research and Consulting).
Understanding these key components and technologies is fundamental to navigating the complex and ever-evolving domain of algorithmic trading. Whether you are a novice trader looking to get started with your first algorithmic trade or an experienced professional seeking to stay ahead of the curve, a deep appreciation for these elements is indispensable.
Strategies and Types of Trades
Algorithmic trading, a method where computers follow a specified set of instructions for placing trades, has revolutionized the trading landscape. The strategies and types of trades available through algorithmic trading are diverse, catering to various asset classes and trading goals.
Common Algorithmic Trading Strategies
Algorithmic trading strategies can be broadly classified into several categories, each with its unique approach and market philosophy. According to Investopedia, key strategies include momentum-based, mean reversion, and market-making strategies.
- Momentum-based Strategies: These strategies follow trends in the market, buying securities that are trending upward and selling those on a downtrend.
- Mean Reversion Strategies: Mean reversion assumes that asset prices will revert to their average over time. These strategies are predicated on the statistical phenomenon of prices to move back towards the mean or average.
- Market-Making Strategies: Market-making algorithms are designed to provide liquidity in the markets by continuously buying at the bid price and selling at the ask price, earning the spread between the two.
Each strategy requires a deep understanding of market dynamics and the capacity to adjust parameters in real-time to maximize profits while mitigating risks. For beginners looking to delve into algorithmic trading, entry-level strategies for new algo traders provides a solid starting point.
Trade Types and Asset Classes
Algorithmic trading spans various trade types and asset classes, enabling traders to diversify their portfolios and manage risks across different markets.
- Foreign Exchange (FOREX): A highly liquid market with a significant volume of trading that benefits from the speed and precision of algorithmic systems.
- Stock Markets: Algorithms can process vast amounts of market data to execute trades on stock exchanges rapidly.
- Exchange-Traded Funds (ETFs): These allow for diversification within an asset class or across different asset classes.
- Bonds: Fixed-income securities are also traded using algorithms, especially to find the best prices in less liquid markets.
- Cryptocurrencies: The volatile nature of digital currencies makes them suitable for high-frequency, algorithm-driven trades.
The table below breaks down the components of the algorithmic trading market, specifically focusing on solutions and services related to different asset classes:
Component | Description | Asset Classes |
---|---|---|
Solutions | Aim to reduce transaction costs and ensure efficient trade execution | FOREX, Stocks, ETFs, Bonds, Cryptocurrencies |
Services | Provide support and maintenance for algorithmic trading systems | FOREX, Stocks, ETFs, Bonds, Cryptocurrencies |
Data adapted from Maximize Market Research
Traders can select the type of trade and asset class based on their capital, risk tolerance, and investment goals. It’s essential to employ the right strategies and to understand the nuances of each trade type and asset class. For those interested in the algorithmic trading of equities, algorithmic trading in equity markets provides additional insights, as does algorithmic trading in forex markets for currency trading enthusiasts.
In conclusion, the global landscape of algorithmic trading offers a plethora of strategies and trade types for various asset classes, enabling traders to execute transactions with speed, accuracy, and efficiency. However, as with any trading endeavor, it is important to be aware of the risks and regulatory framework associated with algorithmic trading.
Impact on Financial Markets
Algorithmic trading has significantly transformed the dynamics of financial markets. By automating the trading process, it has introduced higher levels of efficiency and liquidity, while also affecting price discovery and market volatility.
Market Liquidity and Efficiency
The introduction of algorithmic trading has been instrumental in enhancing market liquidity. It allows traders to execute large orders quickly and with minimal market impact, ensuring that markets remain fluid and functional. This high level of liquidity is beneficial for all market participants, as it provides a stable environment in which to buy and sell securities without causing drastic price changes.
Moreover, algorithmic trading contributes to the overall efficiency of financial markets. With the ability to execute trades at incredible speeds and with great precision, transaction costs are lowered, and traders can capitalize on market opportunities that may be fleeting (Acumen Research and Consulting).
Price Discovery and Volatility
Price discovery is an essential function of financial markets, determining the price at which supply and demand meet. Algorithmic trading has improved this process by facilitating more efficient and accurate order placement. Traders can utilize algorithms to analyze various markets and execute trades at the optimum price, quickly responding to changes in market conditions and ensuring fair value is maintained (Nasdaq).
However, while algorithmic trading has streamlined many aspects of trading, it also has the potential to contribute to market volatility. The speed at which trades can be executed means that significant price movements can occur within fractions of a second. In some cases, this can lead to rapid spikes or crashes in prices if algorithms react simultaneously to market events or signals.
Despite these concerns, the overall impact of algorithmic trading on financial markets is viewed positively by many experts, given its role in lowering transaction costs, improving liquidity, and enhancing the efficiency of market mechanisms. As the global landscape of algorithmic trading continues to evolve, so too will its influence on market behavior and performance. For more insights into the role of algorithmic trading in various asset classes, explore our articles on algorithmic trading in equity markets and algorithmic trading in forex markets.
Risks and Regulatory Landscape
The global landscape of algorithmic trading, while presenting numerous opportunities for efficiency and profitability, also comes with its own set of potential risks and challenges. These must be navigated carefully to maintain the integrity of financial markets and the confidence of investors.
Potential Risks and Challenges
Algorithmic trading, by its nature, can amplify certain market risks. One of the biggest concerns is market volatility, which can lead to substantial financial loss. Traders often employ stop-loss orders to mitigate these risks (Source).
Technical malfunctions represent another significant risk factor. Hardware or software failures, power outages, and connectivity issues can disrupt trading operations. Redundancy measures like backup systems and alternative internet connections are critical preventive strategies (Source).
Risk Factor | Mitigation Strategy |
---|---|
Market Volatility | Stop-loss orders |
Technical Failures | Redundancy measures |
Over-optimization | Out-of-sample testing, Walk-forward analysis |
Data Quality | Data cleaning and validation |
Over-optimization is a challenge that arises when algorithms show promising results on historical data but don’t perform as expected in live markets. Traders address this by employing techniques like out-of-sample testing and walk-forward analysis to ensure the robustness of their algorithms (Source).
The quality of data is another pivotal factor. Inaccurate or incomplete data can lead to flawed trading decisions. Implementing rigorous data cleaning and validation processes is essential to maintain the integrity of trade decisions (Source).
For more information on avoiding common pitfalls, explore our guide on common mistakes in algorithmic trading.
Regulatory Considerations and Compliance
Algorithmic traders must navigate a complex regulatory landscape. Ensuring that trading algorithms comply with relevant financial regulations is not optional but mandatory. Non-compliance can lead to penalties, including financial fines and legal repercussions.
Regulatory bodies across different jurisdictions set guidelines and rules that govern the use of algorithmic trading. These rules may include requirements for transparency, reporting, and monitoring to prevent market abuse and ensure fair trading practices. Traders can collaborate with regulatory consultants and legal advisors to ensure that their trading activities are fully compliant (Source).
Regulatory Aspect | Compliance Strategy |
---|---|
Transparency | Adequate reporting and disclosure |
Reporting Requirements | Timely submission of trading data |
Market Abuse Prevention | Real-time monitoring systems |
To understand the specific regulatory requirements in different markets, traders can refer to our comprehensive analysis of the regulatory framework for algorithmic trading.
As the realm of algorithmic trading evolves, so does the regulatory environment. Keeping abreast of changes and trends is crucial for maintaining compliance and ensuring long-term success in algorithmic trading. For insights into how technology may shape future regulations, consider reading about future technologies impacting algorithmic trading.
The Future of Algorithmic Trading
The landscape of financial trading is continually evolving, and algorithmic trading stands at the forefront of this transformation. As we peer into the future, technological advancements and market trends give us a glimpse of what’s to come in the realm of algorithmic trading.
Technological Advancements
Technological innovation is reshaping the foundation of algorithmic trading. Artificial intelligence (AI) is garnering significant attention for its role in analyzing vast datasets to unearth trading opportunities, learning from historical transactions, and refining strategies. These capabilities allow trading systems to become more sophisticated, although they also raise concerns about unpredictable behaviors and the potential for errors.
AI and machine learning’s integration into trading algorithms is expected to deepen, offering more nuanced and dynamic trading mechanisms that can adapt to changing market conditions with unprecedented speed and efficiency. The application of these advanced technologies is poised to enhance decision-making processes in trading, drive innovation in financial products, and push the boundaries of what can be achieved through automated trading systems.
Groundbreaking advancements extend beyond AI, with high-speed internet and computing power being pivotal contributors to the rise of algorithmic trading. These factors have enabled traders to execute orders at breakneck speeds, with transactions completing in fractions of a second (Nasdaq).
Market Predictions and Trends
The global algorithmic trading market is on an upward trajectory, with a current valuation of USD 15.76 billion in 2023. Projections suggest a Compound Annual Growth Rate (CAGR) of 10.6%, potentially reaching USD 31.90 billion by 2030 (Maximize Market Research). North America remains the epicenter of the algorithmic trading market due to significant investments in trading technologies. Meanwhile, the Asia Pacific region is rapidly emerging as a growth leader, driven by the increasing adoption of algorithmic trading solutions.
As algorithmic trading becomes more prevalent, it now accounts for up to 80% of the total trading volume in the United States and around 50% in other major global markets. This surge is evidence of the growing reliance on automated trading systems, which offer enhanced liquidity, lower transaction costs, and improved price discovery mechanisms within financial markets (Nasdaq).
The future of algorithmic trading also hinges on the development of a robust regulatory framework that can keep pace with the technological advancements. As the market grows, regulatory bodies will play a critical role in ensuring fair and transparent markets, addressing the challenges of market stability, and mitigating risks associated with automated trading (Faster Capital).
As we navigate the global landscape of algorithmic trading, it’s essential to stay informed about the latest advancements, understand the regulatory framework for algorithmic trading, and keep abreast of future technologies impacting algorithmic trading. By doing so, traders, investors, and financial professionals can position themselves to capitalize on the opportunities that algorithmic trading presents while mitigating its inherent risks.